Embodied AI has made significant progress acting in unexplored environments. However, tasks such as object search have largely focused on efficient policy learning. In this work, we identify several gaps in current search methods: They largely focus on dated perception models, neglect temporal aggregation, and transfer from ground truth directly to noisy perception at test time, without accounting for the resulting overconfidence in the perceived state. We address the identified problems through calibrated perception probabilities and uncertainty across aggregation and found decisions, thereby adapting the models for sequential tasks. The resulting methods can be directly integrated with pretrained models across a wide family of existing search approaches at no additional training cost. We perform extensive evaluations of aggre- gation methods across both different semantic perception models and policies, confirming the importance of calibrated uncertainties in both the aggregation and found decisions.
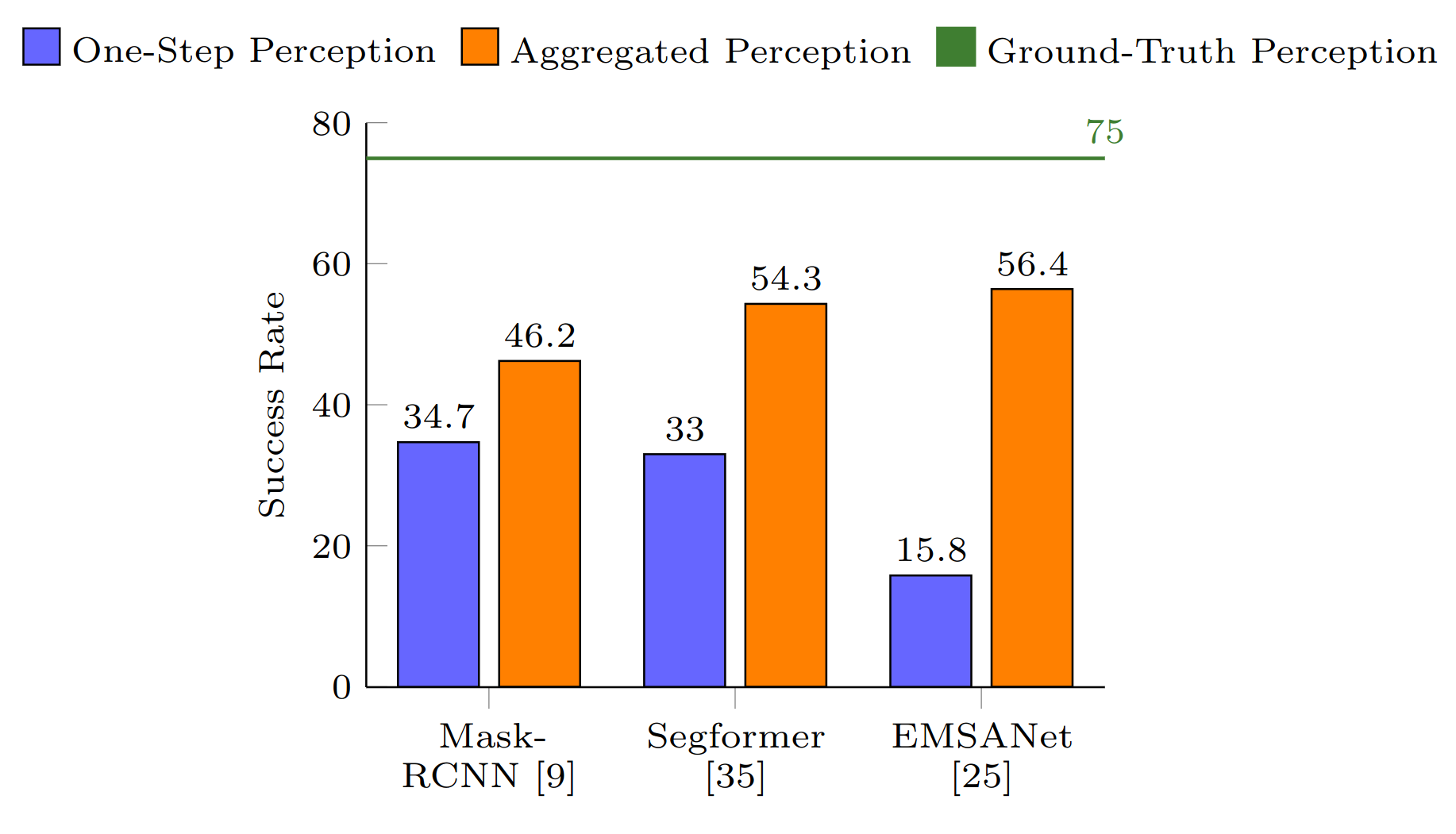
In order to quantify and address these problems, we first evaluate the impact of different semantic perception models and aggregation methods for sequential decision tasks. This differs from pure single-step perception evaluation based on the IoU (Intersection over Union) or precision. In contrast, we measure the results over the full sequence of observations and actions, where early errors may impact or prevent later decisions. The barplot shows the large perception gap to ground truth semantics. While newer models can reduce this gap, we find that temporal aggregation on the perception level is a key to closing the gap. To draw meaningful comparisons, we focus on one of the most used system structures, modular perception - mapping - policy pipelines, in one of the most explored tasks, ObjectNav.